SW 개발
Kotlin, Spring Boot를 이용하여 OpenAI 호환 커스텀 API 서버 제작하기
지단로보트
2024. 10. 21. 01:14
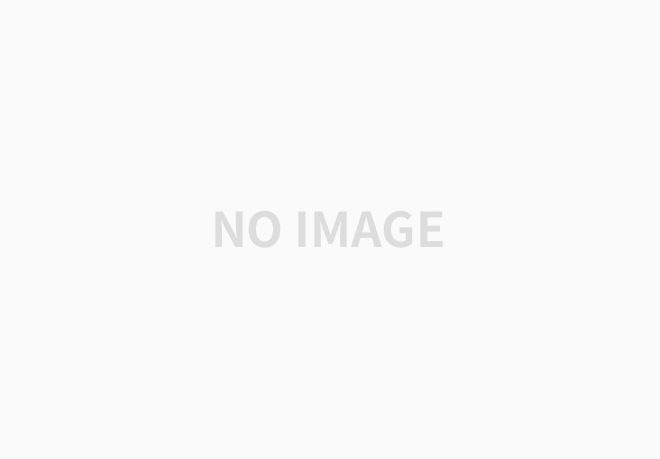
개요
- 2022년 11월 OpenAI가 ChatGPT를 세상에 공개한 후로 OpenAI의 LLM는 사실상의 표준으로 자리 잡았다. LLM 연동을 지원하는 많은 오픈 소스 및 상업 솔루션이 OpenAI의 API와 동일하게 작동하는 OpenAI Compatible API을 지원한다. 이 것의 의미는 많은 기업들이 자사의 내부 보안 환경 및 쓰임새에 맞는 독자적인 OpenAI 호환 서버를 구축하고 운영할 수 있다는 것이다.
- 이번 글에서는 LangChain4j와 Azure OpenAI를 이용하여 OpenAI 호환 서버를 제작하는 방법을 정리했다.
OpenAI Compatible Server 명세
- OpenAI Compatible Server의 핵심은 OpenAI Chat Completion API의 작동을 정확하게 모사하는 것이다. 서버는 아래와 같은 클라이언트의 요청을 받아 LLM의 동작을 수행할 수 있어야 한다.
$ curl -X POST "http://localhost:8080/v1/openai/chat/completions" \
-H "Content-Type: application/json" \
-H "Authorization: Bearer {YOUR_API_KEY}" \
-d '{
"model": "gpt4-o",
"messages": [
{
"role": "user",
"content": "Hello, how are you?"
}
],
"maxTokens": 4096,
"temperature": 0.1,
"stream": true
}'
- 스트리밍 응답의 경우 서버는 Server-Sent Event를 이용하여 각 응답 Chunk를 아래와 같이 클라이언트에 전송할 수 있어야 한다.
{
"id": "unique-emitter-id",
"object": "chat.completion.chunk",
"created": 1633024800,
"model": "gpt4-o",
"choices": [
{
"delta": {
"content": "Hello"
}
}
]
}
- 스트리밍 응답이 완료되면 서버는 Server-Sent Event를 이용하여 아래와 같이 완료 메시지를 클라이언트에 전송할 수 있어야 한다.
[DONE]
프로젝트 생성
Spring Initializr
를 로컬에 설치하고 아래와 같이 신규 프로젝트를 생성한다.
$ sdk install springboot
$ spring init --type gradle-project-kotlin --language kotlin --java-version 21 --dependencies=web openai-comp-demo
$ cd openai-comp-demo
build.gradle.kts
- 프로젝트 루트의
build.gradle.kts
파일에LangChain4j
라이브러리 종속성을 아래와 같이 추가한다.
val langChain4jVersion = "0.35.0"
dependencies {
implementation("dev.langchain4j:langchain4j-core:$langChain4jVersion")
implementation("dev.langchain4j:langchain4j-azure-open-ai:$langChain4jVersion")
}
Creating JsonConfig
- REST API로부터의 응답을 DTO로 변환할
ObjectMapper
빈을 생성한다.
@Configuration
class JsonConfig {
@Bean("objectMapper")
@Primary
fun objectMapper(): ObjectMapper {
return Jackson2ObjectMapperBuilder
.json()
.serializationInclusion(JsonInclude.Include.ALWAYS)
.failOnEmptyBeans(false)
.failOnUnknownProperties(false)
.featuresToDisable(SerializationFeature.WRITE_DATES_AS_TIMESTAMPS)
.modulesToInstall(kotlinModule(), JavaTimeModule())
.build()
}
}
Creating OpenAiCompatibleChatCompletionDTO
- OpenAi Compatible API를 준수하는 DTO를 아래와 같이 제작한다.
data class OpenAiCompatibleChatMessage(
val role: String,
val content: String
)
data class OpenAiCompatibleChatCompletionRequest(
val model: String = "gpt4-o",
val messages: List<OpenAiCompatibleChatMessage>,
val maxTokens: Int = 4096,
val temperature: Float = 0.1f,
val stream: Boolean = false
)
data class OpenAiCompatibleChatCompletionResponse(
val id: String,
val `object`: String,
val created: Long,
val model: String,
val choices: List<OpenAiCompatibleChoice>
)
data class OpenAiCompatibleChoice(
val message: OpenAiCompatibleChatMessage
)
data class OpenAiCompatibleChatCompletionChunk(
val id: String,
val `object`: String,
val created: Long,
val model: String,
val choices: List<OpenAiCompatibleChunkChoice>
)
data class OpenAiCompatibleChunkChoice(
val delta: OpenAiCompatibleDelta
)
data class OpenAiCompatibleDelta(
val content: String
)
Creating OpenAiCompatibleService
- 스트리밍과 비스트리밍 방식을 모두 지원하는 OpenAiCompatibleService 빈을 제작한다.
import com.fasterxml.jackson.databind.ObjectMapper
import dev.langchain4j.data.message.AiMessage
import dev.langchain4j.data.message.UserMessage
import dev.langchain4j.model.StreamingResponseHandler
import dev.langchain4j.model.azure.AzureOpenAiChatModel
import dev.langchain4j.model.azure.AzureOpenAiStreamingChatModel
import dev.langchain4j.model.output.Response
import org.springframework.http.MediaType
import org.springframework.stereotype.Service
import org.springframework.web.servlet.mvc.method.annotation.SseEmitter
import java.time.Instant
import java.util.*
import java.util.concurrent.ConcurrentHashMap
@Service
class OpenAiCompatibleService(
private val objectMapper: ObjectMapper
) {
private val emitters = ConcurrentHashMap<String, SseEmitter>()
fun createChatCompletion(request: OpenAiCompatibleChatCompletionRequest): OpenAiCompatibleChatCompletionResponse {
// LangChain4j에서 제공하는 모든 ChatLanguageModel 구현체를 사용 가능
val chatLanguageModel = AzureOpenAiChatModel.builder()
.apiKey("{your-azure-openai-api-key}")
.endpoint("{your-azure-openai-endpoint}")
.deploymentName("{your-azure-openai-deployment-name}")
.temperature(request.temperature.toDouble())
.maxTokens(request.maxTokens)
.topP(0.3)
.logRequestsAndResponses(true)
.build()
val messages = request.messages.map { UserMessage(it.content) }
val response = chatLanguageModel.generate(messages)
return OpenAiCompatibleChatCompletionResponse(
id = UUID.randomUUID().toString(),
`object` = "chat.completion",
created = Instant.now().epochSecond,
model = request.model,
choices = listOf(
OpenAiCompatibleChoice(
OpenAiCompatibleChatMessage(
role = "assistant",
content = response.content().text()
)
)
)
)
}
fun createStreamingChatCompletion(request: OpenAiCompatibleChatCompletionRequest): SseEmitter {
// LangChain4j에서 제공하는 모든 ChatLanguageModel 구현체를 사용 가능
val streamingChatLanguageModel = AzureOpenAiStreamingChatModel.builder()
.apiKey("{your-azure-openai-api-key}")
.endpoint("{your-azure-openai-endpoint}")
.deploymentName("{your-azure-openai-deployment-name}")
.temperature(request.temperature.toDouble())
.maxTokens(request.maxTokens)
.topP(0.3)
.logRequestsAndResponses(true)
.build()
val emitter = SseEmitter()
val emitterId = UUID.randomUUID().toString()
emitters[emitterId] = emitter
val messages = request.messages.map { UserMessage(it.content) }
streamingChatLanguageModel.generate(messages, object : StreamingResponseHandler<AiMessage> {
override fun onNext(token: String) {
val chunk = OpenAiCompatibleChatCompletionChunk(
id = emitterId,
`object` = "chat.completion.chunk",
created = Instant.now().epochSecond,
model = request.model,
choices = listOf(OpenAiCompatibleChunkChoice(OpenAiCompatibleDelta(content = token)))
)
emitter.send(
SseEmitter.event().data(objectMapper.writeValueAsString(chunk), MediaType.APPLICATION_NDJSON)
)
}
override fun onComplete(response: Response<AiMessage>) {
emitter.send(SseEmitter.event().data("[DONE]"))
emitter.complete()
emitters.remove(emitterId)
}
override fun onError(error: Throwable) {
emitter.completeWithError(error)
emitters.remove(emitterId)
}
})
return emitter
}
}
Creating OpenAiCompatibleController
- 마지막으로 OpenAiCompatibleController 빈을 제작한다.
import org.springframework.http.MediaType
import org.springframework.web.bind.annotation.*
@RestController
@RequestMapping("/v1/openai")
class OpenAiCompatibleController(
private val openAICompatibleService: OpenAiCompatibleService
) {
@PostMapping("/chat/completions", produces = [MediaType.APPLICATION_JSON_VALUE])
fun chatCompletions(
@RequestHeader("Authorization") authHeader: String?,
@RequestBody request: OpenAiCompatibleChatCompletionRequest
): Any {
val apiKey = authHeader?.removePrefix("Bearer ")
// 획득한 API_KEY로 독자적인 커스텀 인증을 적용할 수 있다.
return if (request.stream) {
openAICompatibleService.createStreamingChatCompletion(request)
} else {
openAICompatibleService.createChatCompletion(request)
}
}
}
OpenAI compatible API 작동 테스트
- OpenAI Compatible Server*의 제작이 완료되었다. 제작한 서버를 실행하고, 대표적인 AI 코딩 어시스턴트 도구인 Aider에 환경변수를 지정하면 작동을 확인할 수 있다.
# 프로젝트 실행
$ ./gradlew bootRun
# Aider 환경 변수에 실행 중인 프로젝트의 API 지정
$ export OPENAI_API_BASE=http://localhost:8080/v1/openai/
$ export OPENAI_API_KEY={YOUR_API_KEY}
# Aider 실행
$ aider --model openai/gpt-4o
Aider v0.59.1
Main model: openai/gpt-4o with diff edit format
> Hello, how are you?
Hello! I'm doing well, thank you. How can I assist you with your project today? If you have any specific changes or questions, feel
free to let me know!